안녕하세요 늑대양입니다 😍
이번에 모두의연구소에서 K-디지털 서포터즈로 선정되어 금일부터 12월 23일까지 활동하게 되었습니다.
오늘은 관련 활동의 세 번째 포스팅으로 모두의연구소 MODUPOP에 참석한 경험을 공유드리고자 합니다.
Diffusion 관련 논문 리뷰 1
- 이상윤 님 진행
Introduction
Diffusion models
- Diffusion probabilitstic models
- Score-based models
- DPMs: natural connection with variational approaches
- SBMs: closely related to EBMs (learning an unnormalized density
- Both perspectives are useful for understanding the recently rising iterative methods
Generative modeling
How to construct a flexible pdf?
- Invertible flows - lack of flexibility
- VAEs - surrogate loss
- AR models - sampling speed
- GANs (learning implicit models with min-max game) - training instability
Energy-based models
- Gibbs distribution
- Partition function
- Maximum likelihood training
Score matching
- Due to the intractable partition function, maximum likelihood training is slow.
- Instead, parameterize the log derivative of the density (i.e., score function)
- The partition function disappears as it is not a function of data
- Then, generative modeling is turned into a simple regression task
- Yet, Jacobian trace of the score network is expensive to compute
- 이미지 기준 dimension: 300만
Denoising score matching
- Score matching in the noised data distribution is easier.
- For a Gaussian perturbation kernel
- The above objective is reduced to, which is equivalent to the objective of denoising autoencoders.
- However, we cannot learn the score of the true data distribution
Sampling with Langevin MCMC
- Given a score function, we can sample using Langevin MCMC:
- But there are pitfalls...
Pitfall 1: Inaccurate score estimation in the low-density regions
- The manifold hypothesis tells us that data lies in the low-dimensional manifold.
- As we sample from data distribution, the learned score is accurate only at the data manifold.
- This is problematic as we usually initialize the MCMC with noises.
Pitfall 2: Relative weights
Learning multi-scale score network
- Generative Modeling by Estimating Gradients of the Data Distribution
- Adding Gaussian noise to data can be viewed as "blurring" the data distribution.
- But how to determine the noise strength? >> gradually decrease the variance.
Connection with stochastic differential equations
- A generalizaed framework that unifies the DPMs and SBMs.
- Reveals connection with continuous-time normalizing flows.
- Controllable generation thanks to the modularity of the score networks.
Stochastic differntial equations
- Anderson(1982): A reverse of the diffusion process is also a diffusion process, running backwards in time and given by the reverse-time SDE:
- Can be estimated by learning time conditional score network
DPMs and SBMs are the SDEs
Probability flow ODE
- Generative SDE
- Generative ODE
- PODE yields the same marginal distributions as SDE.
- It can be alseo regarded as a continuous-time flow > exact likelihood computation!
- Fast sampling, smooth interpolation!!
Controllable generation
Controllable generation
Classifier guidance
Classifier-free guidance
- During training, drop out the class label.
- During sampling, mix the conditional and unconditional scores to obtain the classifier gradient.
Blur diffusion
- From a SDE perspective, the generative process of diffusion models can be a reverse-time SDE of various forward stochastic processes
- Can we find the better forward process (and thus the generative process) tailored to a certain data modality of interes (e.g. image)?
Other forward processes
Conclusion
- Diffusion models can be viewed as the discretization of SDEs.
- DPMs and SBMs (Song & Ermon) are the discretizations of VP and VE SDEs.
- Other forward processes can also be considered (e.g. blur).
Q&A
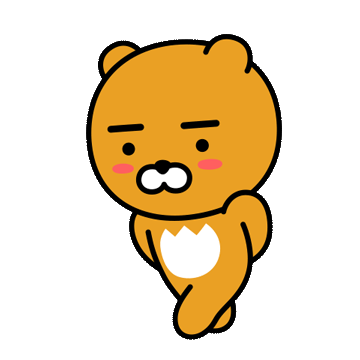
긴 글 읽어주셔서 감사합니다 🤓
'Conference > K-디지털 서포터즈' 카테고리의 다른 글
[모두의연구소][K-디지털서포터즈] MODUCON 2022 - Beyond AI, by Community (0) | 2022.12.23 |
---|---|
[모두의연구소][K-디지털서포터즈] 개발자의 강화학습 (개발자가 강화학습을 취미로 배운다면?) (0) | 2022.12.06 |
[모두의연구소][K-디지털서포터즈] Beauty AI Conference: Data-Centric Vision AI for Beauty (1) | 2022.11.30 |
[모두의연구소][K-디지털서포터즈] NAVER 'HyperCLOVA' 신기술 세미나 (0) | 2022.11.23 |
[모두의연구소][K-디지털서포터즈] 경험공유회: AI 비전공자로 AI 논문쓰기 (0) | 2022.11.22 |